MaskMark - Robust Neural Watermarking for Real and Synthetic Speech
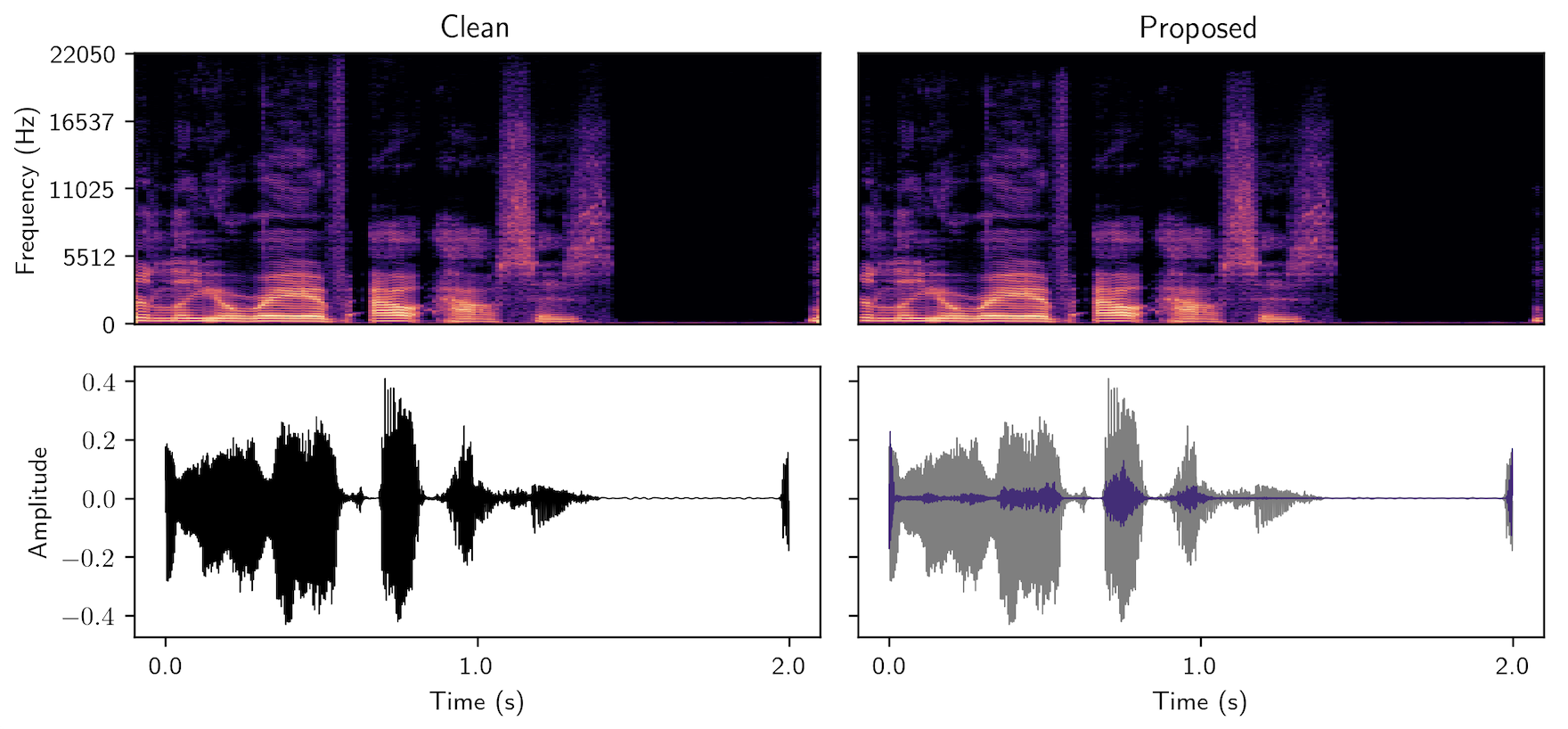
Patrick O'Reilly, Zeyu Jin, Jiaqi Su, Bryan Pardo
Audio Examples
High-quality speech synthesis models may be used to spread misinformation or impersonate voices. Audio watermarking can combat misuse by embedding a traceable signature in generated audio. However, existing audio watermarks typically demonstrate robustness to only a small set of transformations of the watermarked audio. To address this, we propose MaskMark, a neural network-based digital audio watermarking technique optimized for speech.
MaskMark embeds a secret key vector in audio via a multiplicative spectrogram mask, allowing the detection of watermarked speech segments even under substantial signal-processing or neural network-based transformations. Comparisons to a state-of-the-art baseline on natural and synthetic speech corpora and a human subjects evaluation demonstrate MaskMark’s superior robustness in detecting watermarked speech while maintaining high perceptual transparency.
Related publications
[pdf] P. O’Reilly, Z. Jin, J. Su, and B. Pardo, “MaskMark: Robust Neural Watermarking for Real and Synthetic Speech,” in IEEE International Conference on Acoustics, Speech, and Signal Processing (ICASSP), 2024.